

They only show us what a motion skill looks like, not the underlying muscular movements that caused the actor’s muscles to yield that motion.
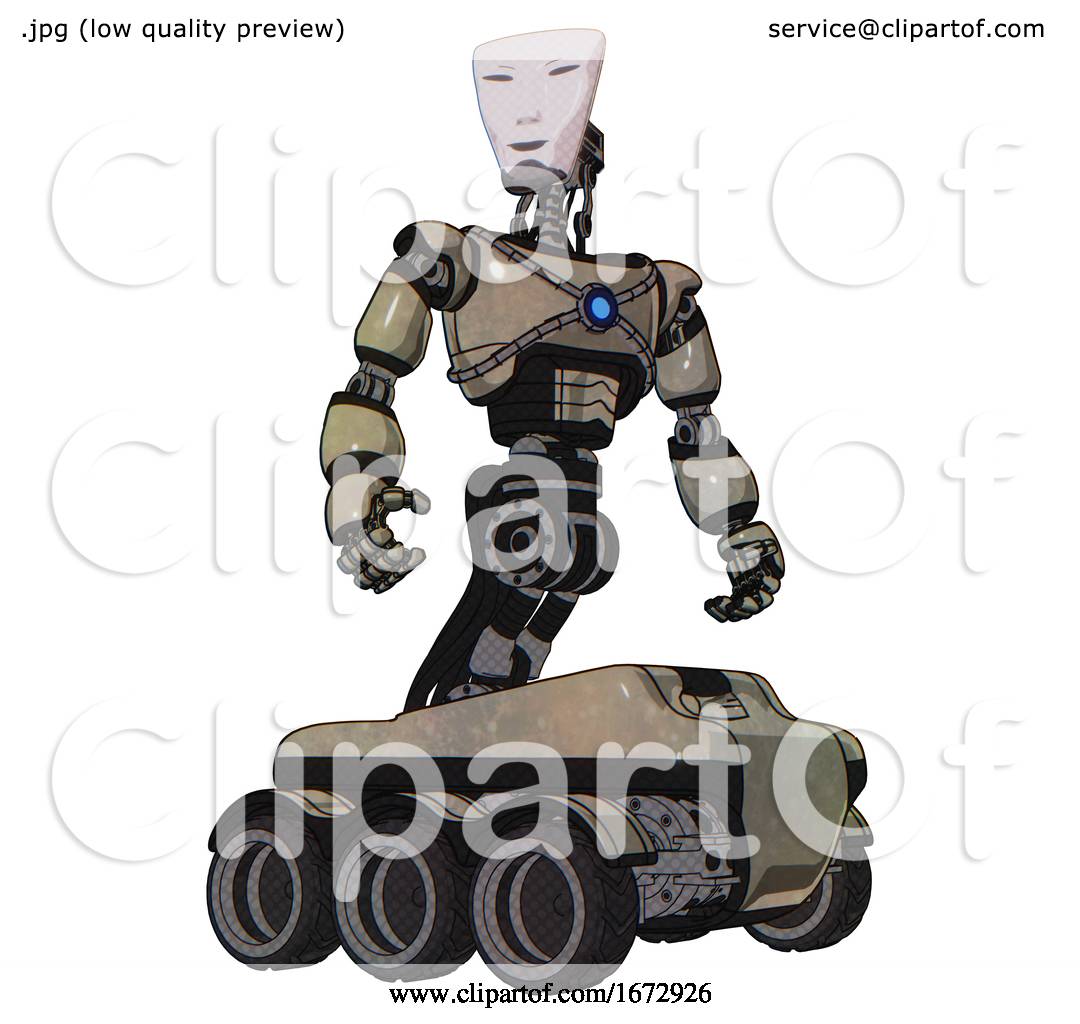
The caveat is that MoCap clips don’t contain all the information necessary to imitate the demonstrated motions on a physical robot or in a simulation that models physical forces.

Why, then, is it so hard to make physical and simulated humanoid robots mimic a person’s movements? Repositories such as CMU Motion Capture Dataset contain hours of clips for just about any common motion of a human body, with visualizations of several examples shown below. On top of this, MoCap data is widely available. Thanks to this, useful information can be extracted from MoCap clips with much less computation than from the much more high-dimensional, ambiguous training data in other major areas of machine learning, which comes in the form of videos, images, and text. Thus, a MoCap clip can be thought of as a very concise and precise summary of an activity’s video clip. It involves recording the motion of several keypoints on a human actor’s body, such as their elbows, shoulders, and knees, while the actor is performing a task of interest, such as jogging. MoCap is an animation technique widely used in the entertainment industry for decades. The prominent avenue for learning locomotive skills is based on using motion capture (MoCap) data. The reason why humanoid control research has been so computationally demanding is subtle and, at the first glance, paradoxical. This will enable advanced research on artificial humanoid control at a fraction of the compute resources currently required. In an effort to level the playing field and make this critical research area more inclusive, Microsoft Research’s Robot Learning group is releasing MoCapAct, a large library of pre-trained humanoid control models along with enriched data for training new ones. Unfortunately, it involves computationally intensive methods, effectively restricting participation to research institutions with large compute budgets. Due to the vagaries of experimentation on physical robots, research in this direction is currently done mostly in simulation. Training AI agents with humanoid morphology to match human performance across the entire diversity of human motion is one of the biggest challenges of artificial physical intelligence. Sophisticated engineering can rein in some of this complexity, but endowing bipedal robots with the generality to cope with our messy, weakly structured world, or a metaverse that takes after it, requires learning. However, even the simplest of these skills can require a fine orchestration of dozens of joints.
HUMANOID AUTOMATRONS FULL
If robots are ever to reach their full potential as an assistive technology, mastery of diverse bipedal motion is a requirement, not a luxury. By mixing and matching a wide range of basic motor skills, from walking to jumping to balancing on one foot, people routinely dance, play soccer, carry heavy objects, and perform other complex high-level motions. What would it take to get humanoid, bipedal robots to dance like Mick Jagger? Indeed, for something more mundane, what does it take to get them to simply stand still? Sit down? Walk? Move in myriads of other ways many people take for granted? Bipedalism provides unparalleled versatility in an environment designed for and by humans.
